Are all genetic algorithms maximization algorithms?
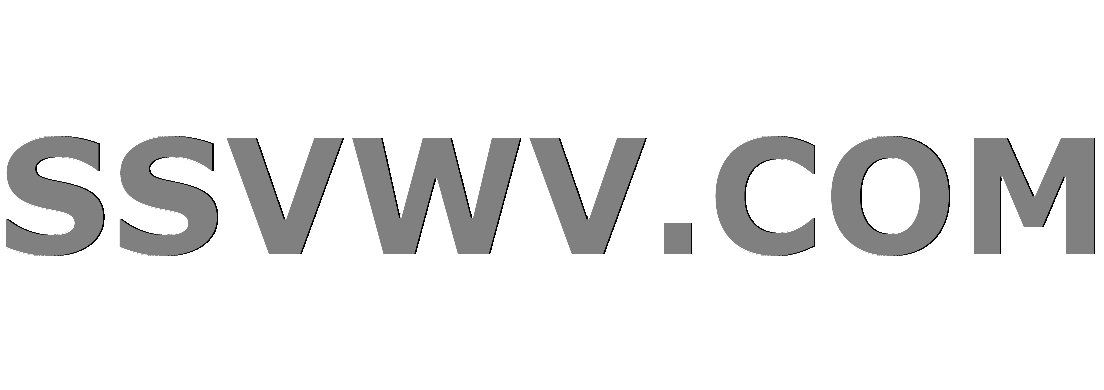
Multi tool use
Are all genetic algorithms maximization algorithms?
I'm not sure if my understanding of maximization and minimization is correct.
So lets say for some function f(x,y,z) I want to find what would give the highest value that would be maximization, right? And if I wanted to find the lowest value that would be minimization?
So if a genetic algorithm is a search algorithm trying to maximize some fitness function would they by definition be maximization algorithms?
The takeaway lesson is: don't get hung up on the max/min terms. Consider a genetic algorithm as a way to explore the search space in order to find local extrema of the "fitness function" (if they exist). These extrema may be local maxima or local minima depending on who you look at things. Then the fitness function changes ("environmental change") and new extrema need to be found..
– David Tonhofer
Apr 23 '14 at 22:48
Note that there's a difference between fitness value and objective value. GAs try to maximise fitness, as they try to evolve fitter individuals. However, this doesn't say that the underlying problem is to minimise or maximise a function. For a minimisation problem, it's just that an individual closer to the minimum is fitter (and thus is of higher fitness). As @DavidTonhofer mentioned, it's easy to convert and usually doesn't matter.
– orange
May 8 '14 at 1:02
To get around this, I think "optimization" is a more frequently used term. Also, it's probably relevant to point out that in a lot of genetic algorithms, particularly those with a diversity maintenance component, the fitness function partially depends on the current population and thus changes over time. This is particularly true in Novelty Search, where fitness is defined entirely by being different from anything that has been found previously. So talking about a maximmum doesn't always entirely make sense.
– seaotternerd
Aug 27 '14 at 3:04
2 Answers
2
So let's say for some function f(x,y,z), I want to find what would give the highest value that would be maximization, right? And if I wanted to find the lowest value that would be minimization?
Yes, that's by definition true.
So if a genetic algorithm is a search algorithm trying to maximize some fitness function would they by definition be maximization algorithms?
Pretty much yes, although I'm not sure a "maximization algorithm" is a well-used term, and only if a genetic algorithm is defined as such, which I don't believe it is strictly.
Generic algorithms can also try to minimize the distance to some goal function value, or minimize the function value, but then again, this can just be rephrased as maximization without loss of generality.
Perhaps more significantly, there isn't a strict need to even have a function - the candidates just need to be comparable. If they have a total order, it's again possible to rephrase it as a maximization problem. If they don't have a total order, it might be a bit more difficult to get candidates objectively better than all the others, although nothing's stopping you from running the GA on this type of data.
In conclusion - trying to maximize a function is the norm (and possibly in line with how you'll mostly see it defined), but don't be surprised if you come across a GA that doesn't do this.
Are all genetic algorithms maximization algorithms?
No they aren't.
Genetic algorithms are popular approaches to multi-objective optimization (e.g. NSGA-II or SPEA-2 are very well known genetic algorithm based approaches).
For multi-objective optimization you aren't trying to maximize a function.
This because scalarizing multi-objective optimization problems is seldom a viable way (i.e. there isn't a single solution that simultaneously optimizes each objective) and what you are looking for is a set of nondominated solutions (or a representative subset of the Pareto optimal solutions).
There are also approaches to evolutionary algorithms which try to capture open-endedness of natural evolution searching for behavioral novelty. Even in an objective-based problem, such novelty search ignores the objective (see Abandoning Objectives: Evolution through the
Search for Novelty Alone by Joel Lehman and Kenneth O. Stanley for details).
While Genetic Algorithms can indeed be used for multiobjective functions, that is not their exclusive purpose: most of the early work (and much ongoing work) is single-objective.
– NietzscheanAI
Jul 2 at 12:43
@NietzscheanAI What you say is true, but the question asked was "Are all genetic algorithms maximization algorithms?". It seems to me that we cannot answer positively since there are important exceptions with practical application.
– manlio
Jul 2 at 15:58
Genetic algorithms are indeed applicable to both maximisation and minimisation problems. They are also applicable to multiobjective problems in which some objectives are to be maximised and some minimised.
– NietzscheanAI
Jul 2 at 20:20
@NietzscheanAI This is also true but the question was "Are ALL genetic algorithms maximization algorithms?" (not "SOME", "MANY"...). I've added to the answer another interesting exception (and there are others).
– manlio
Jul 3 at 9:13
By clicking "Post Your Answer", you acknowledge that you have read our updated terms of service, privacy policy and cookie policy, and that your continued use of the website is subject to these policies.
Well, if your fitness function is real-valued over the search space, just multiply it by -1 and hey presto - maximization becomes minimization (or the reverse).
– David Tonhofer
Apr 23 '14 at 22:26