predict.lm() in a loop. warning: prediction from a rank-deficient fit may be misleading
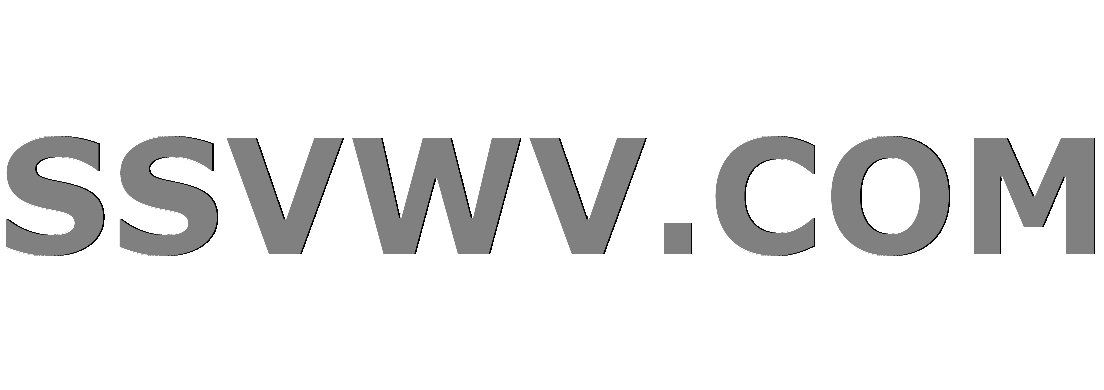
Multi tool use
predict.lm() in a loop. warning: prediction from a rank-deficient fit may be misleading
This R code throws a warning
# Fit regression model to each cluster
y <- list()
length(y) <- k
vars <- list()
length(vars) <- k
f <- list()
length(f) <- k
for (i in 1:k) {
vars[[i]] <- names(corc[[i]][corc[[i]]!= "1"])
f[[i]] <- as.formula(paste("Death ~", paste(vars[[i]], collapse= "+")))
y[[i]] <- lm(f[[i]], data=C1[[i]]) #training set
C1[[i]] <- cbind(C1[[i]], fitted(y[[i]]))
C2[[i]] <- cbind(C2[[i]], predict(y[[i]], C2[[i]])) #test set
}
I have a training data set (C1) and a test data set (C2). Each one has 129 variables. I did k means cluster analysis on the C1 and then split my data set based on cluster membership and created a list of different clusters (C1[[1]], C1[[2]], ..., C1[[k]]). I also assigned a cluster membership to each case in C2 and created C2[[1]],..., C2[[k]]. Then I fit a linear regression to each cluster in C1. My dependant variable is "Death". My predictors are different in each cluster and vars[[i]] (i=1,...,k) shows a list of predictors' name. I want to predict Death for each case in test data set (C2[[1]],..., C2[[k]). When I run the following code, for some of the clusters.
I got this warning:
In predict.lm(y[[i]], C2[[i]]) :
prediction from a rank-deficient fit may be misleading
I read a lot about this warning but I couldn't figure out what the issue is.
3 Answers
3
You can inspect the predict function with body(predict.lm)
. There you will see this line:
body(predict.lm)
if (p < ncol(X) && !(missing(newdata) || is.null(newdata)))
warning("prediction from a rank-deficient fit may be misleading")
This warning checks if the rank of your data matrix is at least equal to the number of parameters you want to fit. One way to invoke it is having some collinear covariates:
data <- data.frame(y=c(1,2,3,4), x1=c(1,1,2,3), x2=c(3,4,5,2), x3=c(4,2,6,0), x4=c(2,1,3,0))
data2 <- data.frame(x1=c(3,2,1,3), x2=c(3,2,1,4), x3=c(3,4,5,1), x4=c(0,0,2,3))
fit <- lm(y ~ ., data=data)
predict(fit, data2)
1 2 3 4
4.076087 2.826087 1.576087 4.065217
Warning message:
In predict.lm(fit, data2) :
prediction from a rank-deficient fit may be misleading
Notice that x3 and x4 have the same direction in data
. One is the multiple of the other. This can be checked with length(fit$coefficients) > fit$rank
data
length(fit$coefficients) > fit$rank
Another way is having more parameters than available variables:
fit2 <- lm(y ~ x1*x2*x3*x4, data=data)
predict(fit2, data2)
Warning message:
In predict.lm(fit2, data2) :
prediction from a rank-deficient fit may be misleading
Thank you for your response.
– Mahsa
Oct 27 '14 at 16:48
Thank you for your response. In cluster 2, C1[[2]] has 130 rows and I have 67 predictors. I found regression function y[[2]]. Then, I used y[[2]] to predict "Death" for all cases in C2[[2]]. C2[[2]] has only 32 rows. Is this the cause of the warning? As I have 32 cases and my regression function has 67 variables? When we use predict.lm, I assumed that we already found the function and the function will be used to predict Death for each case in C2[[2]]. So, I thought it is not important to have more cases than number of predictors. Am I right?
– Mahsa
Oct 27 '14 at 16:55
It is important to have more cases than variables in your model. You can try doing it while having less cases than needed, but you should have in mind that your predictions might be unreliable in that case. That is the reason R gives you a "warning" and not an error. Just to draw your attention. You should be able to get your answers and continue your work even after the warnings (they are not errors), but it would be wise to try simplifying your model.
– Karolis Koncevičius
Oct 27 '14 at 17:05
This warning:
In predict.lm(model, test) :
prediction from a rank-deficient fit may be misleading
Gets thrown from R's predict.lm
. See: http://stat.ethz.ch/R-manual/R-devel/library/stats/html/predict.lm.html
predict.lm
Understand rank deficiency: Ask R to tell you the rank of a matrix:
train <- data.frame(y=c(1234, 325, 152, 403),
x1=c(3538, 324, 382, 335),
x2=c(2985, 323, 223, 288),
x3=c(8750, 322, 123, 935))
test <- data.frame(x1=c(3538, 324, 382, 335),
x2=c(2985, 323, 223, 288),
x3=c(8750, 322, 123, 935))
library(Matrix)
cat(rankMatrix(train), "n") #prints 4
cat(rankMatrix(test), "n") #prints 3
A matrix that does not have "full rank" is said to be "rank deficient". A matrix is said to have full rank if its rank is either equal to its number of columns or to its number of rows (or to both).
The problem is that predict.lm
will throw this warning even if your matrices are full rank (not rank deficient) because predict.lm pulls a fast one under the hood, by throwing out what it considers useless features, modifying your full rank input to be rank-deficient. It then complains about it through a warning.
predict.lm
Also this warning seems to be a catch-all for other situations like for example you have too many input features and your data density is too sparse and it's offering up it's opinion that predictions are brittle.
Example of passing full rank matrices, yet predict.lm
still complains of rank deficiency
predict.lm
train <- data.frame(y=c(1,2,3,4),
x1=c(1,1,2,3),
x2=c(3,4,5,2),
x3=c(4,2,6,0),
x4=c(2,1,3,0))
test <- data.frame(x1=c(1, 2, 3, 9),
x2=c(3, 5, 1, 15),
x3=c(5, 9, 5, 22),
x4=c(9, 13, 2, 99))
library(Matrix)
cat(rankMatrix(train), "n") #prints 4, is full rank, good to go
cat(rankMatrix(test), "n") #prints 4, is full rank, good to go
myformula = as.formula("y ~ x1+x2+x3+x4")
model <- lm(myformula, train)
predict(model, test)
#Warning: prediction from a rank-deficient fit may be misleading
predict.lm sees that the training data has zero information gain, and is tossing out useless features (basically all of them), then says what you've given to it isn't reliable because the model has serious problems.
workaround:
Assuming predict is returning good predictions, you can ignore the warning. predict.lm offers up it's opinion given insufficient perspective and here you are.
So disable warnings on the predict step like this:
options(warn=-1) #turn off warnings
predict(model, test)
options(warn=1) #turn warnings back on
From a numerical aspect, nothing seems misleading.
NA
predict.lm
But perhaps what is numerically correct does not always yield what is statistically or practically favored. If the model matrix is rank-deficient but the predictor matrix is full-rank, some columns of the predictor matrix will be multiplied by 0 coefficients, hence get completely zeroed-out in prediction. This could be what R core worries about.
In the past few hours I tried to conduct an experiment to assess the quality of prediction. In practice there could only be four scenarios:
In machine language terminology, the model matrix is the one associated with training dataset, and the predictor matrix is the one associated with test dataset. The following toy function generates two datasets (each with n
data) for a regression model overall three numeric covariates and an intercept. model.rank1.defect
and predictor.rank1.defect
specify whether we want those matrices to be rank-1 deficient. The reason for using relatively few covariates are commented. The way to achieve deficiency is also commented. Note that the true model is generated on the complete dataset (of 2 * n
data) which has full rank. A noise-to-signal ratio 0.1 is used.
n
model.rank1.defect
predictor.rank1.defect
2 * n
sim <- function (n = 1000, model.rank1.defect = FALSE, predictor.rank1.defect = FALSE) {
## since we only impose rank-1 deficiency, we'd better try small number of parameters
## so that the degree of deficiency is relatively high
## We guess that higher degree of deficiency is,
## the more likely we are going to see "misleading" result
p <- 3
#############################
## similate a model matrix ##
#############################
## generate a full rank model matrix
Xm <- matrix(runif(n * p), n, p)
## make it rank-1 deficient if required
if (model.rank1.defect) {
# take a random column, replace it by the sum of remaining two columns
ind <- sample(p, 1)
Xm[, ind] <- rowSums(Xm[, -ind])
}
#################################
## similate a predictor matrix ##
#################################
## generate a full rank predictor matrix
Xp <- matrix(runif(n * p), n, p)
## make it rank-1 deficient if required
if (predictor.rank1.defect) {
# take a random column, replace it by the sum of remaining two columns
ind <- sample(p, 1)
Xp[, ind] <- rowSums(Xp[, -ind])
}
#########################
## assume a true model ##
#########################
y.true <- 0.5 + rbind(Xm, Xp) %*% rnorm(p)
y <- y.true + rnorm(2 * n, 0, sqrt(0.1 * var(y.true))) ## noise to signal ratio = 0.1
###########################################
## separate training and testing dataset ##
###########################################
train <- data.frame(y = y[1:n], X = I(Xm), y.true = y.true[1:n])
test <- data.frame(y = y[-(1:n)], X = I(Xp), y.true = y.true[-(1:n)])
list(train = train, test = test)
}
Basically we want to see how good / bad the prediction could be (compared with the truth) in four cases. The following function produces scattered plot for four cases, and note that good prediction means that the dots are scattered along y = x
line.
y = x
inspect <- function (seed = 0, n = 1000) {
set.seed(seed)
case1 <- sim(n, model.rank1.defect = FALSE, predictor.rank1.defect = FALSE)
case2 <- sim(n, model.rank1.defect = FALSE, predictor.rank1.defect = TRUE)
case3 <- sim(n, model.rank1.defect = TRUE, predictor.rank1.defect = FALSE)
case4 <- sim(n, model.rank1.defect = TRUE, predictor.rank1.defect = TRUE)
par(mfrow = c(2, 2))
plot(case1$test$y.true, predict(lm(y ~ X, data = case1$train), case1$test), main = "case1", xlab = "true", ylab = "predicted")
plot(case2$test$y.true, predict(lm(y ~ X, data = case2$train), case2$test), main = "case2", xlab = "true", ylab = "predicted")
plot(case3$test$y.true, predict(lm(y ~ X, data = case3$train), case3$test), main = "case3", xlab = "true", ylab = "predicted")
plot(case4$test$y.true, predict(lm(y ~ X, data = case4$train), case4$test), main = "case4", xlab = "true", ylab = "predicted")
}
It is generally a good idea to set a reasonably big n
, say 1000, for clear visualization. You are recommended to try several random seeds, but I will just show the result for 0, 1, 2.
n
inspect(seed = 0, n = 1000)
inspect(seed = 1, n = 1000)
inspect(seed = 2, n = 1000)
Note that for case 1 and case 2, the quality of the prediction appears steadily good. But for case 3 and case 4, it can be good or bad.
y = -x
What does this mean?
So "misleading" just means that the prediction is not always close to truth. So generally we should strive to work with a full-rank model. We probably should do the following:
abline
NA
predict
By clicking "Post Your Answer", you acknowledge that you have read our updated terms of service, privacy policy and cookie policy, and that your continued use of the website is subject to these policies.
The problem is that you get rank-deficient fits. You need to find out which fits give the warning and examine them.
– Roland
Oct 25 '14 at 7:08